On 12 October, Chinese Huawei launched its new Quantum Computing Simulation HiQ Cloud Service Platform (Press Release). On 13 September 2018, the U.S. House of Representatives approved the “H.R. 6227: National Quantum Initiative Act” with $1.275 billion budget from 2019 to 2023 on quantum research. The Chinese government yearly investment in quantum science is estimated to $ 244 million (CRS, “Federal Quantum Information Science: An Overview”, 2 July 2018). The EU Quantum Flagship plans so far to invest €100 million per year, to which national investments must be added. The largest tech companies, be they American, European or Asian, and more particularly Chinese, fund quantum R&D. This heralds the start of a new race for quantum technologies.
- Trump Geopolitics – 1: Trump as the AI Power President
- Uranium for the U.S. Nuclear Renaissance – 2: Towards a global geopolitical race
- The New Space Race (1) – The BRICS and Space Mining
- Uranium for the U.S. Nuclear Renaissance – 1: Meeting Unprecedented Requirements
- Fifth Year of Advanced Training in Early Warning Systems & Indicators – ESFSI of Tunisia
- Towards a U.S. Nuclear Renaissance?
- AI at War (3) – Hyperwar in the Middle east
- AI at War (2) – Preparing for the US-China War?
Indeed, ongoing scientific and technological innovations related to the quantum universe have the potential to fundamentally alter the world as we know it, while also accelerating and even disrupting more specifically the field of artificial intelligence (AI). Advances in quantum technologies have been dubbed the “Second Quantum Revolution”(Jonathan P. Dowling, Gerard J. Milburn, “Quantum Technology: The Second Quantum Revolution”, 13 Jun 2002, arXiv:quant-ph/0206091v1).
In this first article, we shall explain what is this quantum revolution, then narrow it down to where it interacts with AI, indeed potentially accelerating and disrupting current dynamics. This article is aimed at non-quantum physicists, from the analysts to decision-makers and policy-makers, through interested and concerned readers, who need to understand quantum technologies. Indeed, the latter will revolutionise the world in general, AI in particular, as well as governance, management, politics and geopolitics, notably when combined with AI. We shall use as much as possible real world examples to illustrate our text.
We shall explain first where quantum technologies are located, i.e. quantum mechanics. We shall then focus upon these quantum technologies – called the Quantum Information Science (QIS) – concentrating notably on quantum computing and simulation, but also briefly reviewing quantum communication and quantum sensing and metrology. We shall aim at understanding what is happening, how dynamics unfold and the current state of play, while also addressing the question of timing, i.e. when will quantum computing start impacting the world.
Related
Artificial Intelligence – Forces, Drivers and Stakes
The Quantum Computing Battlefield and the Future – Quantum, AI and Geopolitics (2)
Mapping The Race for Quantum Computing – Quantum, AI and Geopolitics (3)
Finally, we shall look at the intersection between the quantum technologies and AI – indeed the emerging Quantum Machine Learning sub-field or even Quantum AI – pointing out possible accelerations and disruptions. We shall therefore highlight why and how quantum technologies are a driver and stake for AI.
Building upon the understanding achieved here, the next articles shall delve more in detail on the potential future impacts on the political and geopolitical world.
From Quantum Mechanics to the new Quantum Technologies
Currently, the principles of quantum mechanics are being newly applied to an array of fields creating the potential for new possibilities in many areas.
Members-only
Download article as pdf (English only)- The Coming Quantum Disruption Artificial Intelligence and Geopolitics – part 1.pdf – 22 pages
Become a member of The Red (Team) Analysis Society.
Quantum mechanics or quantum physics is a scientific discipline, which started at the very beginning of the 20th century, with, initially, work by Max Planck on the colour spectrum (for a rapid and clear summary of the development of the field, read, for example, Robert Coolman, “What Is Quantum Mechanics?“, LiveScience, 26 September 2014).
Quantum mechanics is about “the general expression of the laws of nature in a world made of omnipresent and almost imperceptible particles” (Roland Omnes, Quantum Philosophy: Understanding and Interpreting Contemporary Science, 1999, p.82). This is the reign of the infinitesimally small. Quantum mechanics contributed to a series of scientific changes that stroke at the very heart of the way we understand. As Omnes put it,
“We are loosing the spontaneous representation of the world… common sense is defeated” (ibid.).
Even though common sense was challenged, scientists did not abandon the scientific project and continued their work. Now, the very properties that shocked the scientific community and the new understanding of the world that emerged with quantum mechanics are being used to develop new technologies.
In a nutshell, at the level of the quantum world, we observe a “wave-like nature of light and matter” (Biercuk and Fontaine, “The Leap into Quantum Technology…“, War on the Rocks, Nov 2017). Two resulting properties of quantum systems are then fundamental to the current technological effort, namely superposition and entanglement.
Superposition means that “quantum systems may be (loosely) described as simultaneously existing in more than one place until the system is observed” (Ibid.). Once the system is observed, then the system fixes itself in one place, and one says that “the superposition collapses” (Ibid.).
Entanglement means that “linked particles can be “remotely controlled” no matter how far apart they may be. Manipulate the local partner of an entangled pair and you instantaneously manipulate its entangled partner as well” (Ibid.).
Building notably on these properties, scientists are developing the technological field called the Quantum Information Science (QIS), composed of Quantum sensing and metrology, Quantum communication and Quantum computing and simulation, to which can be added research in Quantum materials. We shall more particularly focus here on Quantum computing.
Understanding Quantum Information Science
Quantum computing and simulation
Quantum computing means harnessing quantum properties, notably superposition and entanglement, “to perform some computation” (CRS, July 2018) in a way that is incredibly faster than what is achieved today by the most powerful High Performance Computing (HPC) capabilities, even the exascale computers, which are currently being built (see Winning the Race to Exascale Computing).
Using quantum computing should be particularly promising for quantum simulations, i.e. “using some controllable quantum system [the quantum computer] to study another less controllable or accessible quantum system” (Georgescu, et al, “Quantum Simulation” 2013). In other words, quantum computing is the best approach to studying and simulating systems located at the quantum level and thus displaying quantum properties.
Quantum computing, a development initiated by security concern
The idea of a quantum computer was developed in 1981 (published in 1982) by American physicist Richard P. Feynman, who thought about using quantum properties to simulate physics and indeed quantum mechanics (“Simulating Physics with Computers“, International Journal of Theoretical Physics, VoL 21, Nos. 6/7, 1982). It was initially mainly of theoretical interest (Simon Bone and Matias Castro, “A Brief History of Quantum Computing”, Imperial College London).
Then, the incredible computing power that a functioning quantum computer could have led to the rising awareness that a “cryptopocalypse” could happen. Indeed, in 1994, mathematician Peter Shor formulated an algorithm, “Shor’s algorithm”, showing that “a quantum computer with a few tens of thousands of quantum bits and capable of performing a few million quantum logic operations could factor large numbers and so break the ubiquitous RSA public key cryptosystem” – the most widely used way to encrypt data transmission (Peter Shor, “Polynomial-Time Algorithms for Prime Factorization and Discrete Logarithms on a Quantum Computer,” 1994, 1995; Seth Lloyd, & Dirk Englund, Future Directions of Quantum Information Processing, August 2016, p.6 ).
It is the 1994 Shor’s findings that created the interest in quantum computing, from which evolved Quantum Technologies (Bone and Castro, Ibid.; Lloyd & Englund, Ibid, Biercuk, “Building The Quantum Future“, video, 2017). The QIS’ birth thus would stem from both the fear of and interest in developing such a quantum computer: Shor’s algorithm would indeed give an incredible security advantage to those benefiting from a quantum computer, as they could break all the codes present, past and future of their ‘competitors’ if these actors use current classical computing capabilities as well as current encryption systems.
What is quantum computing?
Quantum computing is currently being developed. The two main challenges of the field are to develop a usable quantum computer and we are now only at the very early stages of building the hardware, and to learn to program these new computers.
Qubits, hardware and some of the challenges faced
Classical computers store information as 0s and 1s, the bits or binary digits.
For interested and scientifically-minded readers we recommend, among a host of explanations:
Sam Sattel, “The Future of Computing – Quantum & Qubits“, Autodesk.com blog.
Quantum computers use qubits, with which, “you can have zero, one, and any possible combination of zero and one, so you gain a vast set of possibilities to store data” (Rachel Harken, “Another First for Quantum“, ORNL Blog, 23 May 2018).
The short video below (Seeker, 15 July 2018) explains (relatively) simply what are qubits, superposition, and entanglement, as well as the very practical challenges faced to build a quantum computer – i.e. the hardware, such as refrigeration, how to control the state of a qubit and finally how long the information can last inside a qubit, a property called coherence. It then moves to a couple of examples of possible simulations and usage.
For an even better understanding of quantum computing, and although the video is a bit long – 24:15 – we recommend taking the time to watch the very clear, lively and fascinating video by Michael J. Biercuk of the University of Sydney, “Building the Quantum Future“.
Number of qubits, power, and error
Thus, to get a functioning quantum computer, in terms of hardware, you need to have enough qubits to proceed with your computing and to do so in a way where the errors generated by the specificities of quantum computing, notably loss of coherence or decoherence, are not too serious to defeat the whole system. The necessity to consider the errors generated by the quantum system used implies to imagine, create and then implement the best possible quantum error correction, tending towards full quantum error correction. One of the difficulties is that the error correction is also a function of the qubits, which thus multiplies the number of qubits that must be operational.
For example, Justin Dressel of the Californian Institute for Quantum Studies of Chapman University applied Austin G. Fowler et al., “Surface codes: Towards practical large-scale quantum computation” (2012) to Shor’s algorithm using as case study the aim to decrypt a strong RSA encryption using a 2048-bit keys. He calculated that for a quantum computer to meet this goal, its minimum qubit number would be 109. Such a machine would then need to run for 27 hours, to “compare with 6.4 quadrillion years for a classical desktop computer running the number sieve”. Of course, as with classical computers, more qubits would reduce the run-time (for the paragraph, Justin Dressel, Quantum Computing: State of Play, OC ACM Chapter Meeting, 16 May 2018).
Actually, we are still quite far from a 109 qubit computer.
The state of play in terms of qubits processors…
On 16 May 2018, according to Dressel (Ibid.), two main competing implementations (others being in development) are used to obtain physical qubits, and have so far given the following results:
Method 1. Trapped ions – with as best performance
- University of Maryland (UMD)/ Joint Quantum Institute (JQI)*: 53 qubits
Method 2. Superconducting circuits – with as best performance
- Google : 72 qubits – Britlescore
- IBM : 50 qubits
- Intel: 49 qubits (Emile Conover, ScienceNews, 5 March 2018)
- Rigetti Computing : 19 qubits
- CAS-Alibaba Quantum Computing Laboratory: 11 qubits (Rich Brueckner, “Quantum Computing in China: Progress on a Superconducting Multi-Qubits System,” Inside HPC, 4 June 2018)
- UC Berkeley : 10 qubits
… and quantum simulators running on classical computers
Besides the creation of very real quantum computing hardware, we also have the design and development of quantum computing simulators. These allow researchers and scientists to start experimenting with quantum computing and notably to begin learning to program these computers. Indeed, the specificities of quantum computing demand new ways to program these computers.
For example, Atos used its HPC supercomputers to develop Atos Quantum Learning Machine (QLM) with appliances from 30 and 40 Qubits according to power level (Atos QLM Product). Meanwhile, Atos developed “universal quantum assembly programming language (AQASM, Atos Quantum Assembly Language) and a high-level quantum hybrid language” (Ibid.).
Other similar efforts are at work, with, for example, the Centre for Quantum Computation and Communication Technology at the University of Melbourne able “to simulate the output of a 60-qubit machine”, but for “only” an instance of Shor’s algorithm (Andrew Tournson, “Simulation Breaks Quantum Computing World Record“, Futurity, 2 July 2018).
As mentioned in the opening paragraph, Chinese Huawei announced on 12 October that it launched its very first quantum computing simulation platform through its Cloud Service, HiQ (Press release). “The HiQ platform can simulate quantum circuits with at least 42-qubits for full-amplitude simulations” (ibid.), which would make it slightly more powerful than Atos QLM. Of course, performance must be tested by scientists before such conclusions may be drawn with certainty. As Atos, Huawei also developed its quantum programming framework. Unlike Atos’s system, HiQ “will be fully open to the public as an enabling platform for quantum research and education” (Ibid.). We see here emerging two different approaches and strategies to the development of quantum computing, which do and will matter for companies, state actors as well as citizens, as well as for the field. We shall come back to this point in the next article.
When shall we have functioning quantum computers? What is quantum supremacy?
Actually, we already have functioning quantum computers, but their computing power is still weak and they may be considered as prototypes.
Because we already have these prototypes as well as the simulators on classical machines, the current real and relevant question must be transformed into two questions.
1- How powerful does my quantum computer need to be to answer my question or solve my problem?
The first part of our initial timing-related question could be phrased as follows: how powerful does my quantum computer need to be to answer my question or solve my problem?
In other words, the type of computation needed to solve a problem may be more easily and more quickly achieved on a quantum computer with a small number of qubits, but de facto using quantum properties, than on a classical computer, where the very quantum characteristics necessary for solving the problem at hand would demand an enormous HPC, or would just not be feasible. Here, the quantum understanding of the problem under consideration and the algorithm developed become as important, if not more, than the very quantum hardware problem. As a result, current quantum machines and quantum simulations may be considered as already operational.
For example, Vanderbilt University physicist Sokrates Pantelides and postdoctoral fellow in physics Jian Liu, developed detailed quantum mechanical simulations on the atomic scale to help the oil industry know the promise of recovery experiments before they start (Heidi Hall, “Quantum mechanics work lets oil industry know promise of recovery experiments before they start“, Vanderbilt University News, Sep. 27, 2018). They used classical HPC computing facilities at the U.S. National Energy Research Scientific Computing Center of the Department of Energy (DOE). It is likely that if quantum computers had been available to them it would have facilitated their research. Note that the Oak Ridge National Laboratory (ORNL) of DOE has a group focusing on Quantum Information Science – sensing, communicating, computing – and is using Atos Quantum Learning Machine (Atos QLM), a “quantum simulator, capable of simulating up to 40 quantum bits (Qubits)” (Atos Press release, “Atos Quantum Learning Machine can now simulate real Qubits“, 9 April 2018).
As another example, on 4 October 2018, Spanish researchers U. Alvarez-Rodriguez et al. (“Quantum Artificial Life in an IBM Quantum Computer“, Nature, 2018) published the results of their research, according to which they were able to create a quantum artificial life algorithm. Interviewed by Newsweek, Lamata, a member of the scientific team, explained:
“We wanted to know whether emergent behaviors of macroscopic biological systems could be reproduced at the microscopic quantum level,” he said. “What we found in this research is that very small quantum devices with a few quantum bits could already emulate self-replication, combining standard biological properties, such as the genotype and phenotype, with genuine quantum properties, such as entanglement and superposition,” (Hannah Osborne, “Quantum Artificial Life Created for First Time, Newsweek, 11 October 2018).
The life creating simulation was realised using “the superconducting circuit architecture of IBM cloud quantum computer”, with “the IBM ibmqx4 quantum computing chip” (Alvarez-Rodriguez, et al., Ibid.), i.e. using IBM 5 Q, which counts 5 qubits with a maximum qubits connectivity of 4 (“Qubit Quality“, Quantum Computing Report) .
This simulation illustrates perfectly how quantum computing can be both accelerating and disruptive for artificial intelligence, as we shall synthesise in the third part. Indeed, as pointed out in the research paper’s conclusions and prospects, the successful quantum artificial life algorithm could potentially be combined with the new emerging field of quantum machine learning to pursue “the design of intelligent and replicating quantum agents” (Alvarez-Rodriguez, et al., Ibid.). We would reach here potentially a completely new level of AI.
2- When shall we have quantum computers with such a power that classical computers, even the most powerful, are out-powered?
The second part of our question regarding timing could be rephrased as follows: when shall we have quantum computers with such a power that classical computers, even the most powerful, are out-powered, i.e. when will quantum simulations made on classical computers become irrelevant?
This is what Google called achieving “quantum supremacy”, or crossing the “quantum supremacy frontier”, i.e. finding out “the smallest computational task that is prohibitively hard for today’s classical computers” and then going beyond it thanks to a quantum computer (Sergio Boixo, “The Question of Quantum Supremacy“, Google Ai Blog, 4 May 2018). The idea of achieving quantum supremacy is best explained by the following slide from John Martinis’ (Google) presentation “Quantum Computing and Quantum Supremacy” (HPC user Forum, Tuscon, April 16-18, 2018).
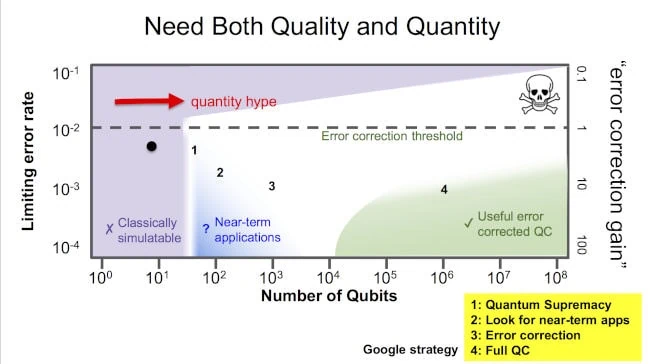
Building upon Google’s slide, Dressel believes we have almost reached “the scale that is no longer possible to simulate using classical supercomputers. The current challenge is to find “near-term” applications for the existing quantum devices” (Ibid.).

However, as improvements in terms of ways to construct quantum simulations on classical machines are also ongoing, then the timeline as well as the numbers of qubits necessary to achieve quantum supremacy could change (Phys.org, “Researchers successfully simulate a 64-qubit circuit“, 26 June 2018; original research: Zhao-Yun Chen et al, “64-qubit quantum circuit simulation“, Science Bulletin, 2018).
Meanwhile, Dressel (Ibid.) also estimates that we can expect chips with one billion qubits in approximately 10-15 years.
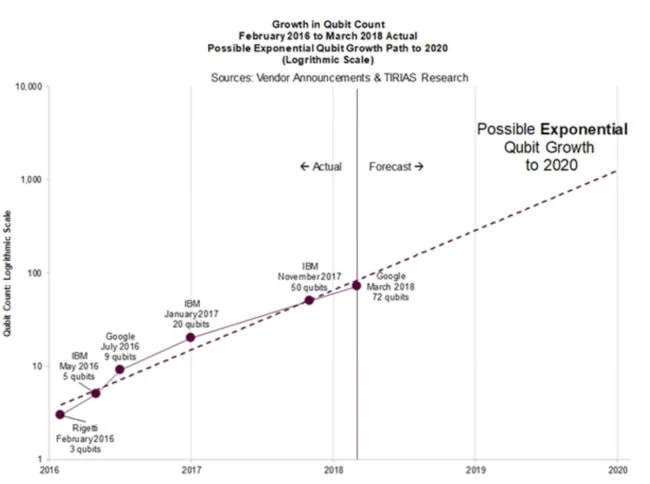
The availability of such a powerful computing power would most obviously be accelerating for AI while completely disrupting the current landscape surrounding the contemporary AI revolution, from the microprocessors developed and used for example in the race to exascale, to the power of those who succeeded in being at the top of the race in terms of classical HPC, but we shall come back to the political and geopolitical implications in the second article of the series.
Quantum communications
As logically evolving from the way quantum technologies were born, quantum communications are mainly concerned with the development of “quantum-resistant cryptography”, as underlined in the U.S. National Strategic Overview for Quantum Information Science, September 2018. If quantum computing can be used to break existing encryption, then quantum mechanics may also be used to protect encryption, notably with quantum cryptography (see phys.org definition) or quantum key distribution (QKD).
Quantum communications is thus about “generating quantum keys for encryption” and more largely, “sending quantum-secure communications (any eavesdropping attempt destroys the communication and the eavesdropping is detected)” (CRS, July 2018, Ibid.).
Quantum sensing and metrology
“’Quantum sensing’ describes the use of a quantum system, quantum properties or quantum phenomena to perform a measurement of a physical quantity” (Degen et al, 2016). Thanks to quantum sensors, we “measure physical quantities such as frequency, acceleration, rotation rates, electric and magnetic fields, or temperature with the highest relative and absolute accuracy.” (Wicht et al. 2018). This video by the UK National Quantum Technology Hub, “Sensors and Metrology“, explains very simply this sub-field.
Applications, including in terms of national security, are numerous, from global positioning systems (GPS), to sub-marines through, for example, improving considerably our understanding of the human brain and of cognition, as explained in the video shown in the last part of the article.
Don’t overstate boundaries
As always, however, if categories between different sub-disciplines are convenient to define fields, focus and explain subject matters, boundaries tend to be porous. Feedbacks with other sub-fields may take place when new discoveries are made. Innovations also emerge at the intersection of the different subfields, as illustrated below with the production of vortices of light in quantum sensing, which then feed into quantum communication – as, for example, unique and identifiable petal patterns can form the alphabet to transmit information (Matthew O’Donnell, “Petal Patterns“, Quantum Sensing and Metrology Group at Northrop Grumman, 17 May 2018).
Accelerating and Disruptive Impacts on AI: the Emergence of Quantum Machine Learning
Related:
When Artificial Intelligence will Power Geopolitics – Presenting AI
Artificial Intelligence and Deep Learning – The New AI-World in the Making
The intersection between the current AI development, which takes place mainly in the area of machine learning and more specifically deep learning and Quantum Information Science is potentially so fruitful that it is giving rise to a new sub-discipline, Quantum Machine Learning.
Below are some of the main areas where research takes place or could take place and where current AI development could be accelerated or disrupted by quantum technologies, while AI possibilities would also impact positively quantum computing.
The first obvious accelerating and potentially disruptive impact quantum computing could have on AI is that once hardware with high number qubits are available, then the (quantum) computing power available also for AI will reach new heights. This is likely to allow for so far impossible to test methodologies, while until now too complex or computing power-hungry algorithms will be developed.
Then, we are likely to see an intensification and multiplication of the development of “creating-AIs”, such as what was done by the combination of evolutionary algorithms and reinforcement learnings by Google Brain Team as well as by scientists at U.S. Department of Energy’s Oak Ridge National Laboratory (ORNL) (see Helene Lavoix, When AI Started Creating AI – Artificial Intelligence and Computing Power, The Red (Team) Analysis Society, 7 May 2018).
Meanwhile, the capacity to see the birth of a third generation AI will be immensely enhanced(see Helene Lavoix $2 Billion for Next Gen Artificial Intelligence for U.S. Defence – Signal ).
As for quantum simulations, some scientists “postulate that quantum computers may outperform classical computers on machine learning tasks.” In that case, Quantum Machine Learning is understood as the field where scientists focus on “how to devise and implement quantum software that could enable machine learning that is faster than that of classical computers” (Jacob Biamonte, et al., “Quantum machine learning“, v2 arXiv:1611.09347v2., May 2018). Quantum Machine Learning algorithms are sought and developed (Ibid., Dawid Kopczyk, “Quantum machine learning for data scientists“, arXiv:1804.10068v1, 5 Apr 2018).
Furthermore, as expected from the second part of this article, the explanations above on QIS, the intersection and feedbacks between quantum systems and AI are also more complex, as far as we can understand and foresee now.
The very challenges involved in quantum computing, i.e. mainly developing the hardware and developing the program and algorithms, could be served by AI. In other words, one would apply the current understanding of AI to quantum computing’s development. Potentially, as we shall proceed through trials and errors, and because of the specificities of quantum computing, AI will evolve, potentially reaching new stages of development. Indeed, for example, as new quantum capabilities are reached, and new simulations become available, new understanding of and approaches to AI may be uncovered.
Also quantum simulations on the one hand, quantum sensing on the other, will produce a new host of big data, which will need AI to be understood.
We can find an example of such a case where AI has been used for these newly available quantum large dataset, which in turn could benefit quantum computing and then most probably AI, in the field of physics in general, superconductivity in particular. On 1st August 2018, Yi Zhang et al. published an article explaining their use of an AI, a specifically designed “array of Artificial Neural Network (ANN)” – i.e. deep learning – on a large body of data, “experimentally derived electronic quantum matter (EQM) image archive”, which allowed for progress in our understanding of superconductivity – notably as far as temperature is concerned, a key challenge in quantum computing (Yi Zhang et al., “Using Machine Learning for Scientific Discovery in Electronic Quantum Matter Visualization Experiments“, 1 August 2018, arXiv:1808.00479v1; for a simplified but detailed explanation, Tristan Greene, “New physics AI could be the key to a quantum computing revolution“, TNW, 19 September 2018).
As a result of this experiment, usage of AI-Deep Learning will most probably increase in physics and more largely in science, while new advances in superconductivity could help towards qubits processors.
Should such a development occur in superconductivity, then this also means that the race to exascale we previously detailed could be disrupted. According to the time when exascale is reached and to the processors used, compared with the time when the new advances in superconductivity can be engineered, as well as when competing quantum processors are available, then the huge computing power finally obtained with exascale as well as the so far developed processor could be more or less obsolete or about to be. The industrial risk should here be carefully estimated and monitored, probably through scenarios as most adapted and efficient methodology. We shall see in the next article the related potential political and geopolitical impacts.
The new types of data gathered by quantum sensing may also enrich our understanding of intelligence in general as with the University of Birmingham project “Quantum Sensing the Brain” (11 June 2018) described in the video below.
This specific quantum sensing achievement may, in turn, thrice change and enrich approaches to AI: first, because we would have had to create new AI-systems to make sense of these specific data, second because these deep learning agents would have had access to new and so far unknown understanding of intelligence, thus would have learned something different enhancing the potential to develop different outputs, and third because the resulting overall new understanding of intelligence could, in turn, generate different and better types of AI.
In the same area, the emerging field of quantum cognition (see Peter Bruza et al., “Introduction to the Special Issue on Quantum Cognition“, Journal of Mathematical Psychology, 23 September 2013; Peter Bruza et al., “Quantum cognition: a new theoretical approach to psychology“, Trends in Cognitive Science, July 2015), now benefiting from quantum simulations, could lead to completely novel approaches to cognition and intelligence. In turn, a disruption of the current status quo in terms of AI around deep learning could occur. Totally new approaches to AI could emerge.
As a result, quantum technologies are indeed a driver as well as a stake for AI.
Although it is still very early in the field of Quantum Information Science, and notably quantum computing and simulations, and even more so in its intersection with AI, considerable innovations have already taken place both in QIS and Quantum AI / Quantum Machine Learning, and the fields are already starting to bear fruits. Many challenges remain, but the efforts endeavoured to overcome these very hurdles could also lead to new breakthrough in both QIS and AI. We could be at the dawn of a real change of paradigm with a whole range of consequences from the already discernible to those difficult to imagine for polities and its actors. It is to these possible impacts we shall turn with the next article.
Featured Image: An image of a deuteron, the bound state of a proton (red) and a neutron (blue). Image Credit: Andy Sproles, ORNL
Notes
*The Joint Quantum Institute (JQI) is actually a group operating “through the work of leading quantum scientists from the Department of Physics of the University of Maryland (UMD), the National Institute of Standards and Technology (NIST) and the Laboratory for Physical Sciences (LPS). Each institution brings to JQI major experimental and theoretical research programs that are dedicated to the goals of controlling and exploiting quantum systems.” (JQI – About). Note that notably through the NIST they will benefit from the 2019 US budget for QIS.
Some references
Alvarez-Rodriguez, U., M. Sanz, L. Lamata & E. Solano, “Quantum Artificial Life in an IBM Quantum Computer“, Nature, Scientific Reports volume 8, Article number: 14793 (2018) – Published: 04 October 2018.
Biamonte Jacob, Peter Wittek, Nicola Pancotti, Patrick Rebentrost, Nathan Wiebe & Seth Lloyd, “Quantum machine learning“, Nature volume 549, pages 195–202, 14 September 2017; revised 10 May 2018 arXiv:1611.09347v2.
Biercuk Michael J., and Richard Fontaine, “The Leap into Quantum Technology: A Primer for National Security Professionals,” War on the Rocks, November 17, 2017.
Biercuk, Michael J., The University of Sydney, “Building the Quantum Future”, Pause Fest, Mar 2, 2017.
Bruza, Peter D., Jerome Busemeyer, Liane Gabora, “Introduction to the Special Issue on Quantum Cognition“, Journal of Mathematical Psychology, 53, 303-305, arXiv:1309.5673v1
Bruza, Peter D., Zheng Wang, Jerome R. Busemeyer, “Quantum cognition: a new theoretical approach to psychology“, Trends in Cognitive Science, Volume 19, Issue 7, July 2015, Pages 383-393.
Congressional Research Service, Federal Quantum Information Science: An Overview, 2 July 2018.
Degen, C. L., F. Reinhard, P. Cappellaro, “Quantum sensing“, Submitted on 8 Nov 2016 (v1), last revised 6 Jun 2017 (this version, v2), arXiv:1611.02427v2 – quant-ph.
Dirjish, Mathew, “Quantum Sensing Platform Now A Reality”, SensorsOnline, July 30, 2018.
Executive Office of the President of the United States, National Strategic Overview for Quantum Information Science, September 2018.
Fowler, Austin G., Matteo Mariantoni, John M. Martinis, Andrew N. Cleland, “Surface codes: Towards practical large-scale quantum computation“, Phys. Rev. A 86, 032324 (2012), arXiv:1208.0928v2
Thank you for this article! It really helped me a lot with my research.